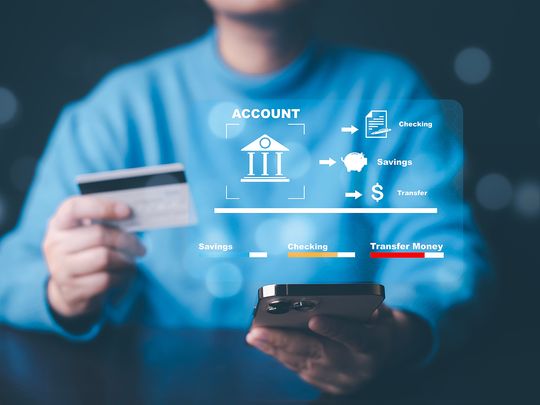
Barclays Bank uses AI for fraud detection. Traders at Bank of America employ an AI tool to uncover hidden market patterns. DBS is leveraging AI to enhance customer service.
Is this the ‘Kodak moment’ for AI in banking?
Having developed at an accelerating pace in recent years, AI is at the centre of a new industrial revolution and is about to transform every sector of the economy. Over 70 per cent of bankers believe that AI will decide the success and failure of banks in the future.
Given that AI runs on data, and banks command vast customer-centric goldmines of data, future banking operations might be re-imagined totally with AI.
Predictive AI or Generative AI?
AI will have a significant impact on the future profitability of banks. This impact will be driven by revenue gains resulting from additional business, a greatly enhanced customer experience and improved pricing. It is estimated that AI can unlock an additional $1 trillion in global banking revenue pools by the end of the decade.
In addition, it can help reduce both operating and risk costs by as much as 30 per cent between now and 2030.
AI is not exactly new to banking. Predictive decision making models based on AI have been used over the past decade for risk monitoring, optimal pricing and product propensity modelling. The rise of generative AI (GenAI) has enriched the broader AI toolkit, enhancing opportunities for banks to create new value with AI. The ability of GenAI models to digest (understand) and generate (converse) in plain language makes AI capabilities more universally accessible.
GenAI and predictive AI are both powerful tools, but they serve very different purposes. As such, using them is not an either-or question. A bank’s AI strategy will need to include both of them going forward, leveraging their respective strengths in different ways.
AI and real benefits
As the race becomes hotter, some banks have embraced innovation and implemented a portfolio of AI use cases to get ahead of the curve. So, what are the use cases that hold out the most potential for the banking industry? And what specific benefits are they expected to yield?
First, in customer-facing front office applications, AI holds considerable promise to drive topline growth and improve customer satisfaction. Generative AI conversational agents are being incorporated in numerous aspects of client interaction.
Credit Mutuel, a large French bank, recently deployed IBM’s AI tool Watson at its branches and achieved a 60 per cent improvement in the speed of service by its 20,000 customer advisors.
Soon, next-best-action and micro-segmentation algorithms will enhance customer experience by predicting financial needs and hyper-personalizing both products and marketing content. JPMorgan Chase is developing IndexGPT, an AI service for investment advice, that aims to redefine investment selection processes.
Second, in the context of middle-office applications, AI is delivering excellent results in detecting and preventing cases of fraud in areas such as anti-money laundering
(AML) and know-your-customer (KYC) compliance. AI is also yielding important benefits for cyber-security, compliance monitoring, credit analysis and loan approval handling.
Banco Santander employs an AI tool called ‘Kairos’ that assesses how corporate clients can be impacted by risk events, making more informed lending decisions.
Finally, back-office applications such as payment processing and IT operations, but also data extraction, document processing and any other processes that lend themselves to automation, are obvious targets for the use of AI.
Many such applications deploy large language models to automate activities such as non-financial reporting like sustainability. Emirates NBD has deployed Microsoft GitHub Copilot to help 2,500 software developers to write, test and deploy new code.
Managing risks
The sheer scale and breadth of these potential benefits – affecting everything from top line to bottom line to customer satisfaction – highlights the importance of acting quickly and resolutely to secure the advantages of AI. Banks need to recruit and develop the right talent, enter the right partnerships and set up robust and scalable IT and big data platforms.
That said, due consideration must also be given to a number of risks and limitations. Regulatory and compliance risks could see banks failing to comply with industry guidelines. Governance risks could pose the threat of serious financial losses. Going forward, these risks must be managed within a comprehensive target operating model.
A model that not just establishes accountability for the development, deployment and maintenance of AI in banking, but also benefits banks and their customers.